.png)
Pricing AI agents: Plans, costs, and monetization models
AI agents are now integral to business operations, demanding a strategic approach to pricing.
This article dives into the essential considerations for pricing AI agents effectively, exploring the nuances that differentiate AI monetization from traditional software models. From variable costs to value-driven expectations, understanding these dynamics is crucial for sustainable growth.
For a quick overview, you can also see our video for some quick examples of how to price AI agents.
Read on to learn:
- The common pricing models emerging for AI agents and their implications
- The key factors that influence AI agent pricing
- How AI agents are reshaping dynamic pricing systems
- Practical frameworks for pricing your AI agent, including real-world examples
- The essential components of an AI billing stack
Let’s start by explaining why AI agents require specific pricing strategies to avoid revenue leaks.
Why AI agents need pricing strategies
The era of free AI experimentation is rapidly closing. As AI agents become integral to business operations, the question is no longer "Will AI charge businesses per user?" but rather, how to price AI to guarantee sustainable growth and value alignment.
Pricing AI agents is not optional; it's a necessity caused by the very nature of the technology. Each prompt, each computation, each API call carries a cost. Ignoring this reality leads to unpredictable expenses and potential budget overruns.
The cost of intelligence: Beyond per-seat models
Traditional software pricing, often based on per-seat subscriptions, fails to capture the unique value proposition of AI agents. These agents are not mere tools; they are autonomous actors, performing tasks that once required human labor.
The infrastructure needed to power them (tokens, compute, and API calls) adds up quickly. Consequently, businesses demand AI agents pricing plans that reflect actual usage and the value delivered. Let’s look at the reasons why billing models for AI agents differ from other models:
- Variable costs = variable billing: Unlike fixed software subscriptions, AI costs fluctuate. Usage spikes, complex tasks, and the sheer volume of operations directly impact expenses. Billing models must adapt to this variability, offering transparency and predictability where possible.
As Jeremy Burton, CEO of Observe, points out, "People get annual budgets and cannot tolerate variability." This means that while consumption-based models may sound good, they can cause budget nightmares.
- Value-driven expectations: Customers expect to pay in proportion to the value they receive. This shift from feature-based to outcome-based pricing is evident in emerging models like per-resolution or per-conversation.
Aligning cost with tangible results becomes crucial for fostering trust and long-term customer relationships. The key to evaluating outcome-based pricing vendors is aligning on success metrics up front.
Note: To further understand the nuances of software monetization strategies, explore our comprehensive usage billing guide and our in-depth post on consumption-based billing.
The 4 most common AI agent pricing models
Unlike traditional SaaS models that charge per seat, AI agents are autonomous doers that perform entire human workflows. This requires new ways to measure value and determine agent price. Here are some of the most common AI agent pricing models.
1. Per-conversation pricing
Charges a fixed rate for each conversation or interaction with the AI agent. Salesforce's Agentforce, for example, charges $2 per conversation.
- Pros: Predictable costs, especially for businesses with fluctuating workloads. Easy to understand and budget for.
- Cons: Can become expensive for continuous, high-volume scenarios. It may not accurately reflect the value of complex resolutions.
2. Outcome-based pricing
The agent price is tied to the successful completion of a task or the achievement of a specific outcome. Chargeflow, for example, claims 25% of recovered chargebacks.
- Pros: Directly aligns costs with results. Incentivizes vendors to deliver value.
- Cons: Defining "success" can be tricky, leading to disputes between vendors and users. Requires clear metrics and governance.
3. "Agentic seat" pricing
In this scenario, AI agents are the "power users." AI agents get their own API access keys and usage quotas.
- Pros: Can be disruptive in areas with many end-users. Offers unlimited work attached to a seat.
- Cons: May not be suitable for use cases with a small number of seats.
4. Token-based consumption
Bills for the number of tokens used by the AI agent, based on the language model's input and output rates.
- Pros: Highly granular and flexible. Users only pay for what they consume.
- Cons: Can be complex to understand and predict costs. Requires careful monitoring of token usage.
What factors influence the pricing of AI agents?
Determining the right pricing for AI agents requires a deep understanding of the various elements that contribute to their operational costs and perceived value. Several key factors come into play:
- Costs to operate: This encompasses the underlying infrastructure costs, including token consumption (the number of tokens processed by the language model), model usage (the computational resources required to run the AI model), and hosting (the costs associated with server space and maintenance).
- Type of task: Simple agents that perform basic tasks will generally be less expensive than complex agents that handle intricate workflows or require advanced reasoning. The complexity of the AI solution directly correlates to its cost.
- Frequency and intensity of use: High-volume usage will naturally incur higher costs. Pricing models must account for fluctuations in demand and offer scalable solutions.
- Support level: Agents that require extensive support, such as ongoing maintenance, updates, or troubleshooting, will typically command higher prices.
- Customization and latency of SLA: Tailored AI agents that are customized to specific business needs and those that offer low-latency service-level agreements will increase the cost.
- Customer size and segment: Pricing strategies may vary depending on the customer segment, with enterprise clients often requiring more robust and expensive solutions than SMBs. These types of users will require firms to implement enterprise billing.
- Integration depth: Agents that operate autonomously and integrate seamlessly with existing systems may be priced differently than those that require human input or manual integration.
Whether or not human interaction is needed to keep the agent operating properly will greatly change the price.
How do AI agents contribute to dynamic pricing systems?
AI agents are reshaping dynamic pricing, going beyond basic supply and demand adjustments. They analyze real-time data, learn user behavior, and personalize value delivery.
In product-led growth (PLG) environments, this translates to AI agents dynamically adjusting their output to match specific customer needs and usage patterns. Pricing AI agents in this way justifies tiered or usage-based billing, where customers pay for the value received.
Personalized value and dynamic agent pricing
AI agents can personalize value. For example, an AI agent handling customer support might offer faster resolution times or more detailed responses to premium users, directly correlating service level with cost.
Beyond adjusting outputs for customers, AI agents themselves can be priced dynamically. Pay-as-you-go models, metered by token, minute, or function, reflect the fluctuating costs of AI operations. Just as a ride-sharing service adjusts prices based on traffic, AI service providers can adjust costs based on computational load or data processing demands.
Why usage-based billing is the future of AI monetization
The shift towards usage-based billing in AI monetization is a logical evolution. Each task an AI agent completes incurs real infrastructure costs — compute, tokens, API calls. A pricing model that reflects actual consumption becomes key.
Usage-based pricing allows vendors to align cost, usage, and revenue with precision. It scales with value delivery; users pay only for what they consume. This approach eliminates the inefficiencies of traditional subscription models, where light users subsidize heavy users.
Plus, it encourages trial and expansion without penalizing those who are just beginning to explore the technology. Companies can offer free tiers or low-cost entry points, knowing that revenue will grow proportionally with usage.
Per-user pricing: When it works and when it breaks
Per-user pricing, a staple of traditional SaaS companies, offers simplicity. For teams and sales operations, it creates predictable costs and straightforward budgeting. It can work well for human-assistive AI tools, where each user benefits from consistent support.
However, per-user pricing breaks down when usage varies wildly between users. A flat fee per person fails to reflect the actual resource consumption. Imagine a sales team: Some individuals might heavily rely on AI-driven lead generation, while others barely touch it. This creates misaligned incentives.
High-use individuals cost more to serve, yet everyone pays the same. The model also ignores the inherent variability of AI costs. Compute, tokens, and API calls fluctuate, making a fixed per-user fee an inaccurate representation of expenses.
Takeaway: In the context of pricing AI agents, a usage-based approach often provides a more equitable and sustainable solution.
How do you price your AI agent? Framework and real examples
Determining the right price for an AI agent is a delicate balance. It requires a deep understanding of the value delivered, the costs incurred, and customer expectations. Here’s a quick look at how to price AI agents, along with examples.
1. Identifying key value metrics
Before setting a price, identify the metrics that truly reflect the agent's value. Is it the number of tasks completed? The time saved for users? Or perhaps the volume of tokens processed?
For example, Decagon measures its customer service agents by resolution rates and customer satisfaction. Salesforce, on the other hand, uses conversations. Define these metrics clearly and make sure they align with customer outcomes.
2. Matching your pricing model to customer expectations
Consider your target audience and their preferred pricing structures. Do they expect a per-seat model, a per-task fee, a flat rate, or a hybrid approach?
Intercom, for instance, offers "digital workforce seats" with free tickets, while Chargeflow uses a success-based model. Aligning your pricing model with customer expectations fosters trust and facilitates adoption. Think about how your customers think about value, and price accordingly.
3. Monitoring infrastructure costs and profitability
Keep a close eye on the underlying infrastructure costs. This includes compute, storage, and token usage. As AI compute prices fluctuate, so too will your expenses.
Microsoft Security Copilot’s $4 per hour model highlights the need for continuous monitoring. Track your profitability and adjust your pricing accordingly to ensure sustainability.
4. Testing thresholds and overages for growth customers
For high-growth customers, consider testing thresholds and overage fees. This allows you to capture the increased value they receive while preventing cost overruns.
For example, you might offer tiered pricing based on usage, with overage fees for exceeding certain limits. This allows for scalability and confirms that your pricing model can accommodate growing demand.
Tips for building flexibility into your AI billing stack
Effectively pricing AI agents and managing revenue requires a robust billing infrastructure that can accurately track usage, apply complex billing logic, and recognize revenue in a compliant manner. Here are the key components you'll need in your AI billing stack:
- Event ingestion for task/token tracking: Accurate usage tracking is paramount. Capture every event, whether it's a completed task, a processed token, or an API call. This data forms the foundation of your usage-based billing. Real-time event ingestion allows you to monitor usage patterns and identify potential anomalies.
- Flexible billing logic (tiers, caps, hybrid): Your billing system must support a range of pricing models, including tiered pricing, usage caps, and hybrid approaches. This flexibility allows you to cater to different customer segments and usage patterns.
- Revenue recognition infrastructure: Make sure your billing system adheres to revenue recognition standards. This process involves accurately tracking when revenue is earned and recognized, which is crucial for financial reporting and compliance. A solid infrastructure prevents revenue leakage and ensures accurate financial projections.
Note: Learn more about billing software requirements here.
Why you should use Orb over traditional billing platforms
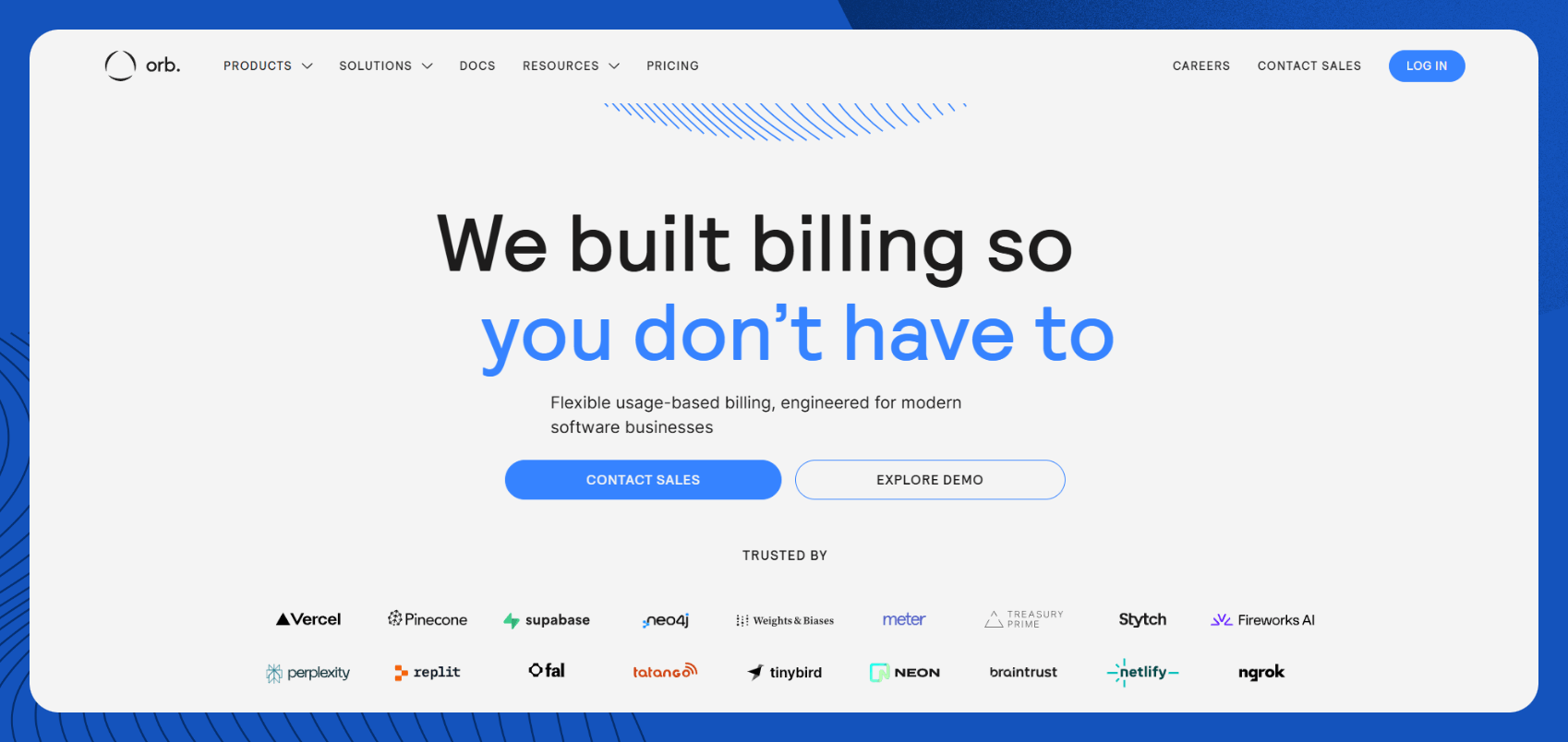
The era of AI agents demands a new approach to pricing and billing. As you've explored in this article, pricing AI agents requires agility, precision, and scalability.
Orb, a done-for-you billing platform, understands these nuances and helps SaaS and GenAI companies transform AI agent pricing into a strategic advantage. It’s designed to support these AI-native pricing models, providing the infrastructure layer for AI billing.
Here's how Orb can help you navigate this evolving landscape:
- AI-native pricing and billing agility: Experiment with pricing models in real time. Orb RevGraph decouples usage data from pricing metrics, enabling easy experimentation and optimization of monetization strategies. Orb SQL Editor allow you to quickly adjust pricing strategies based on usage, task complexity, or token consumption without requiring engineering Lastly, Orb Simulations uses historical data to help you test new pricing models, enabling companies to adapt quickly to evolving AI costs and customer demand.
- Real-time usage tracking and metering precision: Deliver error-free billing and build customer trust with Orb RevGraph. It processes raw event data for accurate, auditable invoices and provides transparent, real-time usage monitoring.
Precisely track tasks, tokens, and API calls, providing accurate billing calculations and preventing revenue leakage. Complete separation of product code from pricing logic provides perfect data accuracy with zero loss.
- Programmable pricing models and invoice generation: Scale your AI agent pricing operations with Orb's extensible API and modular platform. Manage increasing data volumes and complex requirements with built-in revenue analytics and customer usage dashboards.
Orb RevGraph's architecture creates a single source of truth by ingesting all usage data into one unified system. Integrate with your existing financial stack and automate invoice generation.
- Expert partnership for AI monetization: Confidently navigate AI agent pricing with Orb's expert guidance. Get dedicated implementation support, industry expertise, and ongoing optimization assistance.
Benefit from proven migration pathways and regular business reviews for smooth strategy adoption. As a leader in usage-based pricing, Orb acts as a trusted guide for companies navigating AI-native monetization.
Ready to start monetizing AI agents the right way? Explore our flexible pricing options and find a plan that works for you.
Ready to solve billing?
